Machine Learning (ML)
What do you mean by Machine Learning?
Machine Learning (ML) is a subset of artificial intelligence (AI) that focuses on the development of computer algorithms and models that can learn and make predictions or decisions without being explicitly programmed. It is based on the idea that machines can learn from and adapt to data, enabling them to perform tasks and make accurate predictions.
How does Machine Learning work?
Machine Learning algorithms operate by using statistical techniques to analyze and identify patterns in large sets of data. These algorithms learn from the data and adjust their model parameters to make accurate predictions or decisions. The process involves training the algorithm on a labeled dataset, evaluating its performance, and refining it until it achieves the desired accuracy.
What is known about Machine Learning?
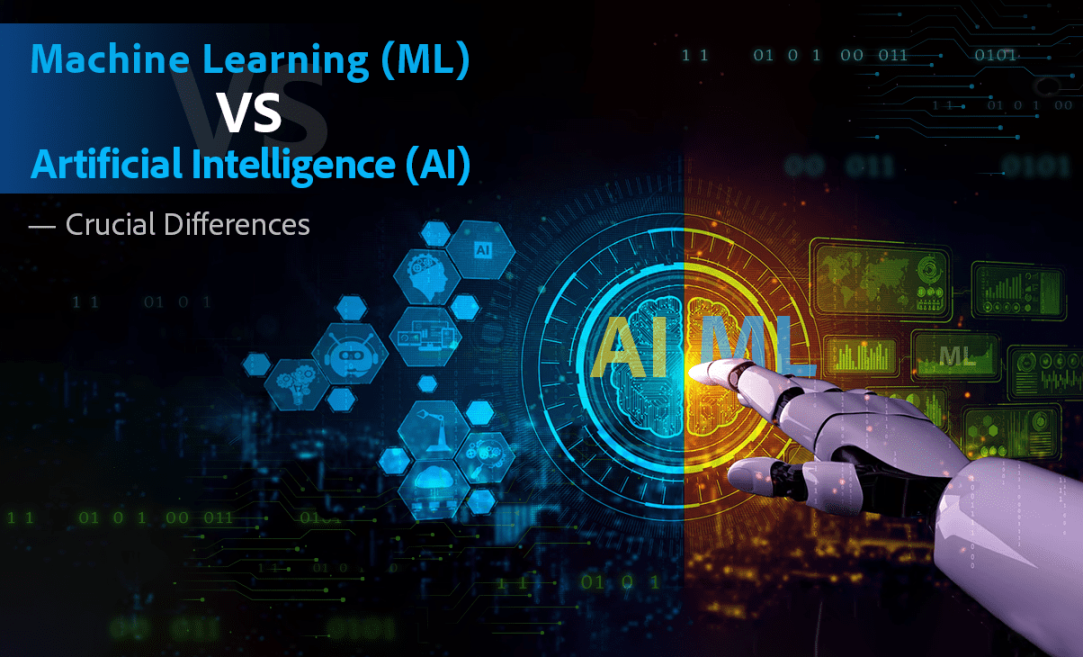
Machine Learning has been around for several decades, but recent advancements in computing power and the availability of vast amounts of data have fueled its rapid growth. It has found applications in various fields, including image and speech recognition, natural language processing, recommendation systems, fraud detection, and autonomous vehicles.
Solution provided by Machine Learning
Machine Learning offers solutions to complex problems that were traditionally difficult to solve using traditional programming approaches. It can analyze and process massive amounts of data, enabling it to identify patterns, make predictions, and automate decision-making processes. ML algorithms can also continuously learn from new data, improving their performance over time.
Information about Machine Learning

Machine Learning is broadly categorized into three types: supervised learning, unsupervised learning, and reinforcement learning. Supervised learning uses labeled data to train models and make predictions. Unsupervised learning identifies patterns and structures in unlabeled data. Reinforcement learning involves a system learning through trial and error interactions with an environment to maximize rewards.
Common Machine Learning algorithms include linear regression, decision trees, random forests, support vector machines, k-nearest neighbors, and neural networks. Each algorithm has its strengths and weaknesses, making them suitable for different types of problems.

Machine Learning also heavily relies on data preprocessing, feature engineering, and model evaluation techniques. Data preprocessing involves cleaning, transforming, and normalizing data to make it suitable for analysis. Feature engineering focuses on selecting or creating relevant features to improve model performance. Model evaluation techniques help assess the accuracy and performance of ML models.
Machine Learning Conclusion
Machine Learning is revolutionizing various industries and driving advancements in artificial intelligence. Its ability to analyze and learn from data has paved the way for intelligent systems, automation, and personalized experiences. With ongoing research and development, Machine Learning is expected to become even more powerful and influential in the future.
Machine Learning FAQs
1. Is Machine Learning the same as artificial intelligence?
No, Machine Learning is a subset of artificial intelligence. While AI encompasses a broader range of technologies and concepts, Machine Learning specifically focuses on algorithms and models that can learn from data.
2. Can anyone learn Machine Learning?
Yes, anyone with an interest in Machine Learning can learn it. There are numerous online courses, tutorials, and resources available that cater to beginners as well as experienced professionals.
3. What are some common applications of Machine Learning?
Machine Learning finds applications in various domains, including healthcare, finance, e-commerce, cybersecurity, and autonomous systems. It is used for medical diagnosis, fraud detection, personalized recommendations, autonomous driving, and much more.
4. Are Machine Learning models always accurate?
No, the accuracy of Machine Learning models depends on various factors, including the quality of data, preprocessing techniques, feature selection, and the chosen algorithm. It is essential to evaluate and refine models to improve their accuracy.
5. Is Machine Learning replacing human jobs?
Machine Learning has the potential to automate certain tasks and processes, but it is not meant to replace humans. Instead, it aims to augment human capabilities, improve efficiency, and enable humans to focus on more complex and creative endeavors.